Object recognition and passerby counting using AI
The people in the image are whited out to protect their privacy, while the AI counts the number of pedestrians walking on the street. In addition to people, it can also recognize various objects such as cars and bicycles using machine learning. By utilizing AI to automatically detect age and gender and count the number of people 24 hours a day, 365 days a year, this data could serve as valuable market information for the AI era.
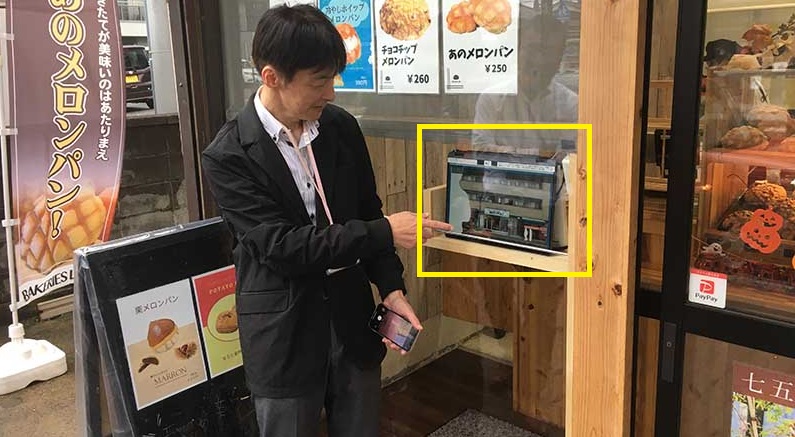
For example, if this technology is installed in a store, it can automatically count the number of passersby throughout the year. By analyzing factors such as weather, the number of passersby, and past sales data, it can predict future sales with high accuracy. These precise AI predictions contribute to optimizing store staffing and purchasing quantities, addressing SDG-related issues such as food loss, and reducing greenhouse gas emissions associated with disposal and incineration.